Real-Time Analytics for Fraud Detection in Financial Services
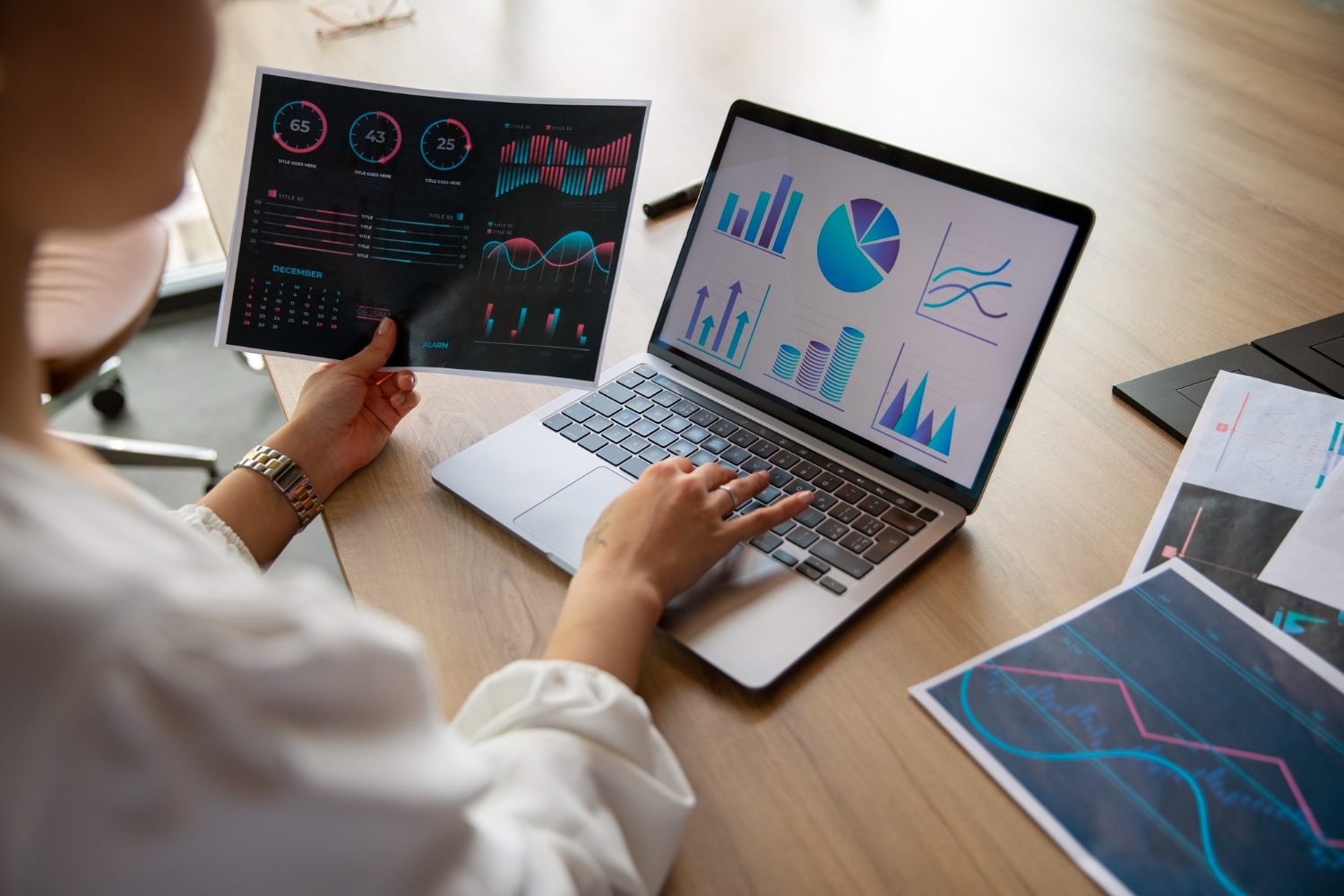
Financial fraud is becoming increasingly sophisticated, leveraging technology to exploit vulnerabilities in banking and financial systems. From credit card fraud and identity theft to insider threats and synthetic identity scams, the risks are growing. To combat these threats, financial institutions are turning to real-time analytics, AI, and machine learning to detect and prevent fraud before it causes significant harm.
The Evolving Nature of Financial Fraud
Digital transformation has changed how financial services operate, offering speed and convenience to customers. However, it has also opened up new avenues for fraudsters. As per a report by PwC in 2023, more than 51% of financial organizations reported experiencing fraud in the past two years, with cybercrime being the most common type.
Real-time analytics helps institutions analyze massive volumes of transactional data instantly. This speed enables them to detect anomalies, flag suspicious behavior, and act before fraudulent transactions are completed.
What is Real-Time Fraud Detection?
Real-time fraud detection refers to the continuous monitoring of transactions as they occur. This process uses streaming analytics and machine learning models to identify irregular behavior patterns—such as unusual spending locations, time-based inconsistencies, or sudden transaction spikes.
The goal is not just to detect fraud but to do so fast enough to block the transaction or trigger alerts before the damage is done. Unlike traditional batch processing systems, real-time analytics evaluates transactions within milliseconds.
How Data Analytics Powers Real-Time Detection
Real-time fraud detection is made possible by advanced data analytics services that integrate various data sources, process large-scale datasets, and deploy models that evolve over time. These systems learn from historical data and adapt to new fraud tactics, ensuring constant vigilance.
By implementing data analytics solutions, financial firms can:
-
Analyze customer behavior patterns in real time
-
Score transaction risks based on multiple variables
-
Identify coordinated fraud attempts across multiple accounts
-
Automate decision-making without human intervention
This allows for highly accurate fraud detection, reducing both false positives and missed threats.
Key Technologies Behind Real-Time Fraud Detection
Several cutting-edge technologies work in unison to enable financial institutions to detect fraud in real time:
1. Machine Learning & AI
These models analyze historical fraud data and learn to recognize subtle signs of suspicious activity. They continuously improve as they process more data.
2. Streaming Analytics Platforms
Platforms like Apache Kafka or Apache Flink are used to ingest and process data in real-time. They enable high-throughput, low-latency analytics essential for fraud detection.
3. Graph Analytics
Fraud rings often operate through connected entities. Graph analytics help identify relationships and detect organized fraud by mapping account connections and transaction patterns.
4. Behavioral Biometrics
By tracking how users interact with their devices—keystroke rhythm, mouse movements, or touch pressure—systems can detect anomalies that signal potential fraud.
Real-World Use Cases in Financial Services
Credit Card Fraud Prevention
Banks use real-time analytics to monitor card transactions against customer profiles. If a transaction deviates significantly from normal behavior, it is flagged or blocked instantly.
AML (Anti-Money Laundering)
AI-driven analytics helps institutions detect layering or structuring activities that may indicate money laundering attempts. These activities are typically hard to spot in traditional audits.
Insider Threat Detection
Using data analytics services, companies can analyze employee activity logs and communication trails to identify patterns that suggest malicious insider actions.
According to IBM’s Cost of a Data Breach 2023 report, financial firms using AI and analytics were able to reduce fraud detection time by 27% and cut associated losses by up to 35%.
Benefits of Real-Time Analytics for Financial Fraud
1. Faster Detection and Response
With data analytics solutions running in real-time, financial services can react within seconds to block suspicious activity—minimizing customer impact.
2. Improved Customer Trust
Preventing fraud before it happens helps preserve customer confidence, a critical factor in the finance sector where trust is paramount.
3. Regulatory Compliance
Real-time analytics systems provide detailed audit trails and risk scoring that help comply with financial regulations such as PCI-DSS, GDPR, and KYC/AML standards.
4. Cost Reduction
By automating fraud detection and minimizing false positives, organizations can lower operational costs related to manual reviews and customer claims.
Challenges in Adoption
Despite its advantages, implementing real-time fraud detection systems presents challenges such as:
-
Integration with legacy infrastructure
-
Managing data privacy and governance
-
The need for skilled data scientists and engineers
-
Constantly evolving fraud techniques requiring adaptive models
To overcome these, financial firms must invest in scalable, cloud-based data analytics services and foster a culture of continuous innovation.
The Road Ahead
The global fraud detection and prevention market is expected to grow from $28.8 billion in 2023 to $74.6 billion by 2030, according to Fortune Business Insights. As fraudsters adopt more advanced tools, the pressure on financial institutions to act in real-time will only intensify.
Looking forward, we can expect greater adoption of explainable AI, federated learning, and privacy-preserving analytics to balance security with compliance and customer trust.
Conclusion
Real-time analytics has become an essential weapon in the fight against financial fraud. Financial institutions can no longer rely solely on periodic checks or manual intervention. Instead, they must embrace intelligent, real-time data analytics solutions that can detect, interpret, and act on threats instantly.
By adopting modern data analytics services, financial organizations can not only protect their bottom line but also deliver a safer and more seamless experience to their customers.
- Art
- Causes
- Crafts
- Dance
- Drinks
- Film
- Fitness
- Food
- Spiele
- Gardening
- Health
- Home
- Literature
- Music
- Networking
- Other
- Party
- Religion
- Shopping
- Sports
- Theater
- Wellness