Federated Learning: The Future of Private AI Training
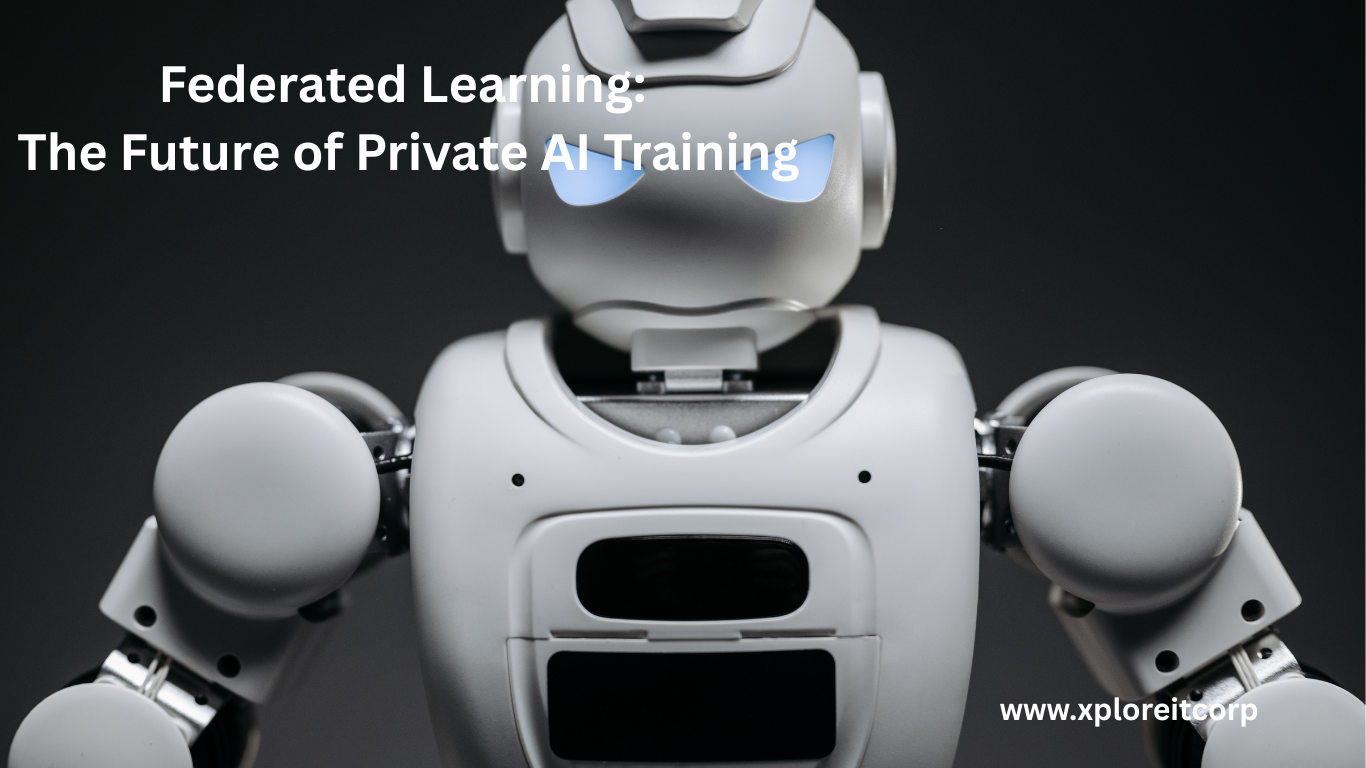
Today's businesses are scrambling to discover alternate ways to harness the power of artificial intelligence without jeopardizing sensitive data, despite the fact that data privacy concerns have never been greater. The innovative method that is rapidly changing the landscape of private AI training is called federated learning. One of the main problems with developing AI nowadays is that models can be trained on dispersed data sets without ever becoming centralized thanks to a distributed training paradigm. Most professionals choose to enroll in higher level specialized Artificial Intelligence Course in Coimbatore at Xplore IT Corp courses in order to learn these techniques, as more and more organizations and businesses realize the importance of data sovereignty. Aside from being purely technological, the shift away from privacy-blind AI approaches is an existential reexamination of how machine learning should be conducted in the modern world when privacy is paramount.
Comprehending Federated Learning: A Revolution in Thinking
The conventional centralized model of AI training differs significantly from federated learning. In traditional machine learning solutions, data collected from many sources is transported to a central location where model training is conducted. Sensitive information must leave its original location, which inevitably leads to privacy and security concerns when centralization occurs.
By bringing the model to the data instead of the data coming to the model, federated learning reverses this dilemma. Prior to being deployed on local devices or servers, the model is trained centrally. The local data is then used to train the local model. The information is returned to improve the core model, not the data. In the face of increasingly stringent regulations, this astounding response is already generally regarded as the Future of Private AI Training since it allows businesses to benefit from collective intelligence while maintaining data confidentiality.
Federated Learning Methods
The following steps make up a traditional federated learning process:
Distribution of the First Model: A first model is installed on the central server and sent to servers or units that are willing to volunteer.
Local Training: Using its local data, each device trains the model individually, generating unique model updates.
Aggregation of Updates: Local model updates are sent to the central server, but not the raw data.
Model Improvement: To update the global model, the central server retrieves local modifications.
Iteration: The devices receive the revised global model, and the procedure is repeated.
Private data is safely stored on local devices, where it should be, thanks to this one cycle at a time approach.
The Reasons Federated Learning Will Shape Private AI Training in the Future
Numerous factors point to federated learning as the future of private AI training when considering the field of artificial intelligence.
1. Enhanced Protection of Privacy
Federated learning offers an armory of defense in a world when data breaches are making the front page of newspapers more frequently. Businesses drastically reduce the attack surface that is susceptible to potential data breaches by reducing raw data down to a local level. Implementing federated learning in privacy-sensitive domains including private communications, financial services, and healthcare is particularly beneficial due to its structure.
2. Adherence to the Regulation
Organizations are facing increasing pressure to manage data responsibly as a result of international frameworks, such as the CCPA in California and the GDPR in the European Union. By limiting data migration across jurisdictional boundaries, federated learning provides a compliance path. The technicalities of federated learning deployment and these regulatory frameworks are likely to be covered in an AI course in Coimbatore.
3. Reduced Data Transfer Expenses
Massive data transfers over networks are quite costly and require a lot of bandwidth. Because model updates are shared and orders of magnitude smaller than raw data, federated learning keeps these costs low. The more data sets and model sizes there are, the more important this becomes.
4. Usage of Edge Devices
Distributed computing has never-before-seen possibilities thanks to the proliferation of intelligent gadgets. Without centralization, federated learning allows the models to learn from actual usage patterns by taking advantage of the edge computing capabilities. For experts who have finished a artificial intelligence course in coimbatore and are interested in IoT applications and mobile AI systems, this is a really useful tool.
5. Working Together to Learn Across Organizations
The most concerning aspect of federated learning may be its ability to facilitate collaboration without data sharing. Businesses can now more effectively work on AI possibilities without encroaching on one other's data area, even if they were never willing to aggregate their sensitive data. The competitiveness between industries is changing as a result of such collaborative potential.
Technical Foundations of Federated Education
The future of private AI training also includes understanding the technical underpinnings that allow federated learning to take place:
Protocols for Safe Aggregation
Federationed learning systems prefer secure aggregation protocols to prevent indirect data leakage through model updates. These cryptographic algorithms offer an extra degree of privacy protection by allowing the central server to compute averaged updates without seeing individual contributions.
Integrating Differential Privacy
The majority of federated learning solutions make use of differential privacy, a mathematical idea that adds precisely constructed noise to data or model updates. Once again supporting privacy guarantees, this has the effect of discouraging the reconstruction of a single data point while retaining statistical relevance.
Communication Effectiveness
Given that federated learning operates on networks with varying bandwidth capacities, communication effectiveness might become critical. Advanced deployments use techniques like dynamic scheduling, update sparsification, and model compression to reduce the transmission bottleneck.
Heterogeneity Management
Federated learning, as opposed to centralized approaches, must address heterogeneous data distribution among devices. Expert algorithms must learn robust models in the "non-IID" (non-independent and identically distributed) problem domain when data is heterogeneous. AI students in Coimbatore study these issues and their solutions as part of their course work.
Applications in Industry Showing the Prospects for Private AI Training
Federated learning's transformative potential is already being utilized in a number of industries:
Medical Care: Federated Medical Research
Federated learning is being used by hospitals worldwide to develop diagnosis models without transferring patient data between facilities. While ensuring patient confidentiality and compliance, this procedure enables unmatched collaboration. Large healthcare facilities are working with Coimbatore-based organizations that offer AI course in coimbatore to train their staff in these privacy-preserving techniques.
Smartphone Technology: Tailored User Experience
Without transferring user behavior data to a central place, federated learning is used by app developers and device manufacturers to improve voice interpretation, content recommendations, and predictive text. This method ensures customisation based on privacy.
Financial Services: Networks for Fraud Detection
To create effective anti-fraud systems that learn from transaction patterns across several firms without disclosing private client financial information, banks and other financial institutions employ federated learning. Data boundaries are strictly enforced to ensure joint protection at the ecosystem level.
Production: Optimal Predictive Maintenance
In remote facilities, manufacturing companies use federated learning to improve predictive maintenance models without exchanging operating data that can contain sensitive information about equipment setups or production processes.
Telecommunications: Optimizing Networks
By using federated learning to analyze usage trends globally without storing user-specific data, telecom companies can improve network performance. Providing responsive network management without compromising privacy is made possible.
Difficulties on the Path to Broad Adoption
Although federated learning has promise as the future of private AI training, there are several issues that researchers and practitioners are attempting to resolve:
The heterogeneity of the system
Devices with varying processing power, storage capacities, and communication patterns must be supported by federated systems. Because of this heterogeneity, designing the system and algorithm is more difficult. In order to address these real implementation challenges, higher-level courses like the AI course in Coimbatore usually require hands-on experience.
Models' susceptibilities to poisoning
An adversarial participant tries to pollute the global model with tainted updates, a technique known as model poisoning, and the decentralized environment of federated learning creates possible attack surfaces for such attacks. Anomaly detection systems and resilient aggregation strategies are crucial defenses.
Barriers to Communication
Communication can still be a bottleneck source even in cases where updates are extremely effective, particularly in situations with low bandwidth or inadequate connectivity. Recent research aims to get around these limitations by using asynchronous learning protocols and ultra-efficient update encoding.
Difficulties with Statistics
The non-IID nature of scattered data poses statistical difficulties that impair model efficacy or convergence. The creation of model resilience requires highly complex algorithms to deal with distribution differences.
The Path Ahead: Prospects for Federated Learning
Some intriguing trends are anticipated when federated learning develops further as the future of private AI training:
Cross-Silo and Cross-Device Cooperation
The region is making a distinction between "cross-silo" federation of organizational data centers and "cross-device" federation of several consumer gadgets. The finest people to handle the opportunities and challenges presented by each paradigm are those who have taken an artificial intelligence course in Coimbatore.
Federated Learning in the Vertical Direction
While most implementations focus on horizontally partitioned data (same examples, different features), vertical federated learning can handle situations when the same examples from multiple parties have different features. A new kind of collaborative possibility among complementing datasets is represented by this.
Transfer Learning Federated
By combining federated and transfer learning, models can leverage previously learned knowledge and adjust locally to data distributions. This hybrid approach is likely to accelerate learning in situations of scarcity.
Integration with Blockchain
In order to provide transparent audit trails of model updates without compromising data privacy, later systems combine federated learning with blockchain technology. This integration resolves trust issues in multi-stakeholder federations.
Multiple-Task Federated Education
Advanced deployments enable the federation to learn numerous related jobs at the same time, achieving optimal knowledge sharing without compromising data boundaries. Low-resource environments greatly benefit from the optimization.
Organizational Considerations for Implementation
When considering federated learning as the future of private AI training, businesses should consider a number of implementation-related factors:
Needs for Infrastructure
Federated learning reduces the need for centralized data storage, but it still requires effective infrastructure to disseminate the model, receive updates, and combine updates. Businesses must consider how technically capable they are of facilitating such a distributed strategy.
Knowledge of Privacy Engineering
Specialized knowledge in privacy engineering is necessary for a successful deployment. In order to develop capabilities internally rather than hiring outside expertise, many organizations invest in reskilling current personnel through training, such as AI training in Coimbatore.
Structures of Governance
Data use, model ownership, and participation rules in federated systems must be specified using well-defined governance frameworks. These ought to be in line with the organization's values and the requirements of the law.
Aligning Incentive Programs
In federations with several organizations from various disciplines, incentive programs must be carefully designed to encourage involvement and high-caliber contributions from all stakeholders. The intricacies of implementation may not always be as important as these economic considerations.
Tracking Performance
Strong monitoring systems must be put in place by organizations to keep an eye on model performance, spot potential threats, and confirm that the federation functions as intended. The privacy assurances that make federated learning worthwhile must be upheld by these monitoring systems while providing visibility.
Routes for Education to Federated Learning Proficiency
As federated learning increasingly represents The Future of Private AI Training, universities are developing cutting-edge programs to build expertise in this emerging field:
Academic Initiatives
Leading computer science colleges now offer specialized courses in machine learning that protects privacy, with federated learning as one of the main topics of study. Scholarly curricula provide the fundamental theory required to progress the field.
Expert Education
Experiential training in the implementation of federated learning systems is offered to corporate leaders through executive training programs like the Artificial Intelligence Course. These programs integrate both practical implementation training and theoretical instruction.
Open Source Groups
There are currently active ecosystems for open-source federated learning platforms like PySyft and TensorFlow Federated. These ecosystems provide practitioners at all levels with top-notch educational resources and collaborative tools.
Certifications for the Industry
Industry certifications are emerging to verify proficiency in privacy-preserving AI techniques, even though the field is still in its infancy. They enable companies to identify competent experts who can lead federated learning initiatives.
conclusion
Federated learning is at the forefront of private AI training in the future and offers a convincing solution to the seemingly conflicting demands of data utilization and privacy protection. Adoption is continuing to pick up speed as businesses across a range of sectors, including healthcare, banking, retail, and technology, realize the strategic advantages of privacy-preserving AI methods. In our increasingly privacy-conscious world, the method is a trailblazing technology since it may promote collective intelligence without requiring data sharing.
Investments in education are crucial for such experts who want to lead this change. Modules like the AI module in Coimbatore provide the specific training required for the appropriate implementation and responsible usage of such technologies. Federated learning competencies will become increasingly important for firms as regulatory scrutiny increases and consumers increase their privacy demands.
Serious organizational, governance, and technical obstacles stand in the way of comprehensive federation. However, the benefits—better privacy protection, compliance, reduced data transmission costs, and unprecedented collaborative potential—are so alluring that progressive companies will find the trip worthwhile.
Federated learning is a bright spot for the future, a time when privacy and AI progress must coexist. By bringing the model to the data rather than the other way around, this approach has to turn AI design upside down and make privacy a top priority rather than an afterthought. There is no limit to the possibilities of individuals who are prepared to embrace this new paradigm. To discover more about how you may attend professional training in federated learning and other state-of-the-art methods and contribute to the privacy-preserving AI revolution, cick here.
- Art
- Causes
- Crafts
- Dance
- Drinks
- Film
- Fitness
- Food
- Giochi
- Gardening
- Health
- Home
- Literature
- Music
- Networking
- Altre informazioni
- Party
- Religion
- Shopping
- Sports
- Theater
- Wellness